
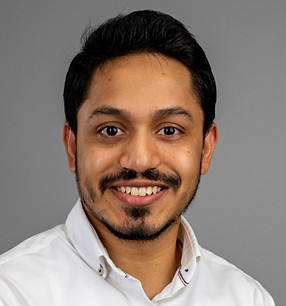
Muhammad Maaz
Ph.D. Computer Vision
MBZUAI
I am a Ph.D. Candidate in the Computer Vision Department at MBZUAI, advised by Dr. Salman Khan and Prof. Fahad Khan.​​​​
My research is focused on developing multimodal large language models (MLLMs) for detailed video understanding, multimodal reasoning and long-video understanding.​​​​​
Highlights
[Apr 26, 2024] LLaVA++ is released!
| Code | HF Collection | HF Demo | Google Colab |
[Jun 24, 2024] I have joined Meta as Research Scientist Intern with Christoph Feichtenhofer.
Publications
VideoMathQA: Benchmarking Mathematical Reasoning via Multimodal Understanding in Videos
| Paper | Website | Hugging Face |
Hanoona Rasheed, Abdelrahman Shaker, Anqi Tang, Muhammad Maaz, Ming-Hsuan Yang, Salman Khan, Fahad Shahbaz Khan
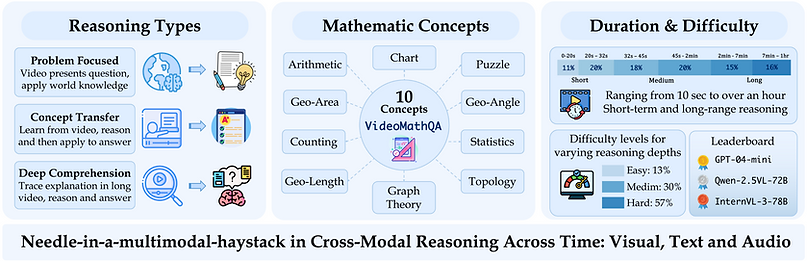
VideoMathQA is a benchmark designed to evaluate mathematical reasoning in real-world educational videos. It requires models to interpret and integrate information from three modalities, visuals, audio, and text, across time. The benchmark tackles the needle-in-a-multimodal-haystack problem, where key information is sparse and spread across different modalities and moments in the video.
PerceptionLM: Open-Access Data and Models for Detailed Visual Understanding
| Paper | Code | Hugging Face |
Muhammad Maaz*, Jang Hyun Cho*, Andrea Madotto*, Effrosyni Mavroudi*, Triantafyllos Afouras*, Tushar Nagarajan*, Yale Song*, Tengyu Ma*, Shuming Hu*, Suyog Jain, Miguel Martin, Huiyu Wang, Hanoona Rasheed, Peize Sun, Po-Yao Huang, Daniel Bolya, Nikhila Ravi, Shashank Jain, Tammy Stark, Shane Moon, Babak Damavandi, Vivian Lee, Andrew Westbury, Salman Khan, Philipp Krähenbühl, Piotr Dollár, Lorenzo Torresani, Kristen Grauman, Christoph Feichtenhofer
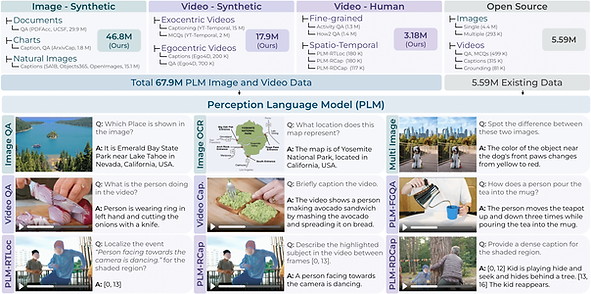
PerceptionLM (PLM) is a family of open and fully reproducible models to facilitate research in vision-language modeling (VLM). It is powerful enough to compete with the latest state-of-the-art VLMs such as InternVL3 and QwenVL2.5, while using fully open data. We also release the largest spatiotemporally annotated video dense captioning and fine-grained video QA datasets to ever exist.
VideoGPT+: Integrating Image and Video Encoders for Enhanced Video Understanding
| Paper | Code | Hugging Face | Benchmark |
Muhammad Maaz, Hanoona Rasheed, Salman Khan, Fahad S. Khan
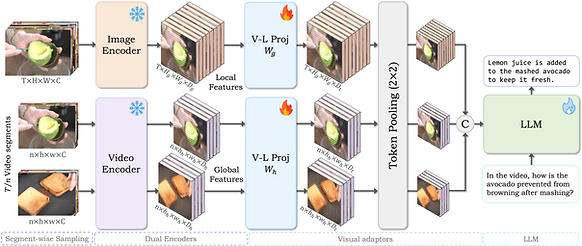
VideoGPT+ integrates image and video encoders to leverage detailed spatial understanding and global temporal context, respectively. It processes videos in segments using adaptive pooling on features from both encoders, enhancing performance across various video benchmarks.
Video-ChatGPT: Towards Detailed Video Understanding via Large Vision and Language Models
| ACL 2024 | | Paper | Code | Hugging Face | Benchmark |
Muhammad Maaz*, Hanoona Rasheed*, Salman Khan, Fahad S. Khan

Video-ChatGPT is a video conversation model capable of generating meaningful conversation about videos. It combines the capabilities of LLMs with a pretrained visual encoder adapted for spatiotemporal video representation. It also presents the first quantitative benchmarks to evaluate video-based conversational models.
Mobile-VideoGPT: Fast and Accurate Video Understanding Language Model
| Paper | Code | Hugging Face |
Abdelrahman Shaker, Muhammad Maaz, Chenhui Gou, Hamid Rezatofighi, Salman Khan, Fahad Shahbaz Khan
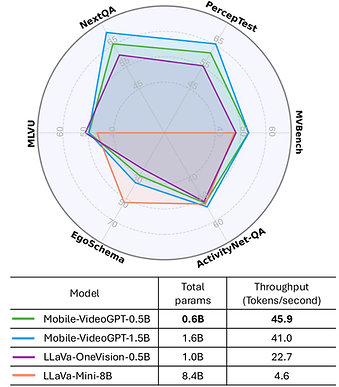
Mobile-VideoGPT is an efficient multimodal model designed to operate with fewer than a billion parameters. Unlike traditional video MLLMs, Mobile-VideoGPT consists of lightweight dual visual encoders, efficient projectors, and a small language model (SLM), enabling real-time inference on resource-constrained platforms. To further improve efficiency, we present an Attention-Based Frame Scoring mechanism to select the key-frames, along with an efficient token projector that prunes redundant visual tokens and preserves essential contextual cues. We evaluate our model across well-established six video understanding benchmarks (e.g., MVBench, EgoSchema, NextQA, and PerceptionTest), and our results show that Mobile-VideoGPT-0.5B can generate up to 46 tokens per second while outperforming existing 0.5B-parameter competitors.
GLaMM: Pixel Grounding Large Multimodal Model
| CVPR 2024 | | Paper | Code | Hugging Face | Dataset | Website |
Hanoona Rasheed*, Muhammad Maaz*, Sahal Shaji, Abdelrahman Shaker, Salman Khan, Hisham Cholakkal, Rao Muhammad Anwer, Eric Xing,
Ming-Hsuan Yang, Fahad S. Khan
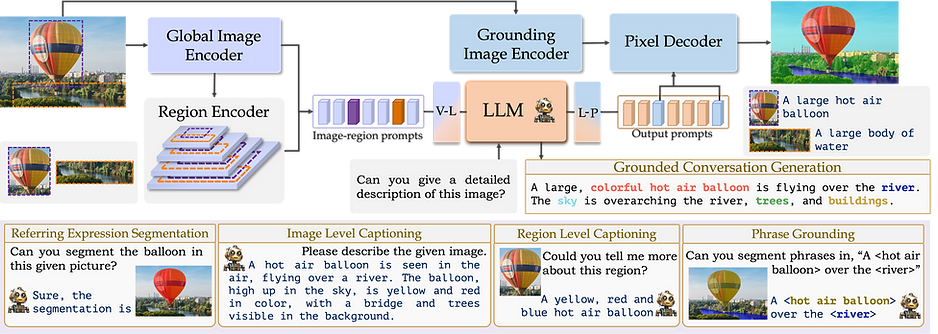
Grounding Large Multimodal Model (GLaMM) is an end-to-end trained LMM which provides visual grounding capabilities with the flexibility to process both image and region inputs. This enables the new unified task of Grounded Conversation Generation that combines phrase grounding, referring expression segmentation and vision-language conversations. Equipped with the capability for detailed region understanding, pixel-level groundings, and conversational abilities, GLaMM offers a versatile capability to interact with visual inputs provided by the user at multiple granularity levels (objects, object parts, attributes, relationships and holistic scene).
PALO: A Polyglot Large Multimodal Model for 5B People
Hanoona Rasheed, Muhammad Maaz, Abdelrahman Shaker, Salman Khan, Hisham Cholakal, Rao M. Anwer, Tim Baldwin, Michael Felsberg, Fahad S. Khan
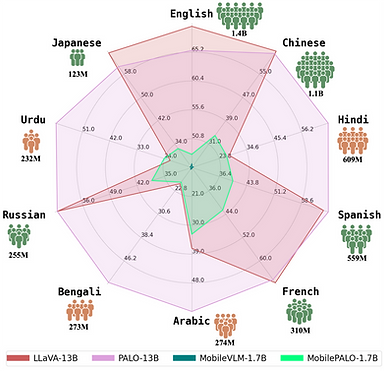
PALO is the first mutlilingual Large Multimodal Model that offers visual reasoning capabilities in 10 major languages, including English, Chinese, Hindi, Spanish, French, Arabic, Bengali, Russian, Urdu, and Japanese, that span a total of ~5B people (65% of the world population). Our approach involves a semi-automated translation approach to adapt the multimodal instruction dataset from English to the target languages using a fine-tuned Large Language Model. We train models across three distinct scales i.e., 1.7B, 7B, and 13B parameters to demonstrate the scalability of our training pipeline. The models demonstrate good performance on low-resource languages, e.g., Hindi, Arabic, Bengali, and Urdu, without compromising its high-performance on high-resource languages e.g., English, Chinese, French, and Spanish.
Hanoona Rasheed, M. Uzair Khattak, Muhammad Maaz, Salman Khan, Fahad Khan

In this work, we show that a simple Video Fine-tuned CLIP (ViFi-CLIP) baseline is generally sufficient to bridge the domain gap from images to videos. Our qualitative analysis illustrates that the frame-level processing from CLIP image-encoder followed by feature pooling and similarity matching with corresponding text embeddings helps in implicitly modeling the temporal cues within ViFi-CLIP. Such fine-tuning helps the model to focus on scene dynamics, moving objects and inter-object relationships. For low-data regimes where full fine-tuning is not viable, we propose a ‘bridge and prompt’ approach that first uses fine-tuning to bridge the domain gap and then learns prompts on language and vision side to adapt CLIP representations.
M. Uzair Khattak, Hanoona Rasheed, Muhammad Maaz, Salman Khan, Fahad Khan
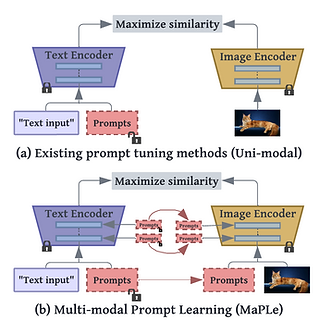
In this work, we propose to learn prompts in both vision and language branches of pretrained CLIP for adapting it to different downstream tasks. Previous works only use prompting in either language or vision branch. We note that using prompting to adapt representations in a single branch of CLIP (language or vision) is sub-optimal since it does not allow flexibility to dynamically adjust both representation spaces on a downstream task. To this end, we propose Multi-modal Prompt Learning (MaPLe) for both vision and language branches to improve alignment between the vision and language representations. Our design promotes strong coupling between the vision-language prompts to ensure mutual synergy and discourages learning independent uni-modal solutions.
Bridging the Gap between Object and Image-level Representations for Open-Vocabulary Detection | NeurIPS 2022 | | Paper | Code | Website |
Hanoona Rasheed*, Muhammad Maaz*, M. Uzair Khattak, Salman Khan, Fahad Khan

In this work, we propose to solve the Open-vocabulary detection (OVD) problem using pretrained CLIP model, adapting it for object-centric local regions using region-based distillation and image-level weak supervision. Specifically, we utilize high-quality class-agnostic and class-specific object proposals via the pretrained multi-modal vision transformers (MViT). The class-agnostic proposals distill region-specific information from CLIP and class-specific proposals allow us to visually ground large vocabularies. We also introduce a region-conditioned weight transfer method to get complementary benefits from both region-based distillation and image-level supervision.
Class-agnostic Object Detection with Multi-modal Transformer
| ECCV 2022 | | Paper | Code | Presentation |
Muhammad Maaz*, Hanoona Rasheed*, Salman Khan, Fahad Khan, Rao M.Anwer, Ming-Hsuan Yang

In this work, we explore the potential of the recent Multi-modal Vision Transformers (MViTs) for class-agnostic object detection. Our extensive experiments across various domains and novel objects show the state-of-the-art performance of MViTs to localize generic objects in images. We also develop an efficient and flexible MViT architecture using multi-scale feature processing and deformable self-attention that can adaptively generate proposals given a specific language query.
SwiftFormer: Efficient Additive Attention for Transformer-based Real-time Mobile Vision Applications | ICCV 2023 | | Paper | Code |
Abdelrahman Shaker, Muhammad Maaz, Hanoona Rasheed, Salman Khan, Ming-Hsuan Yang, Fahad S. Khan
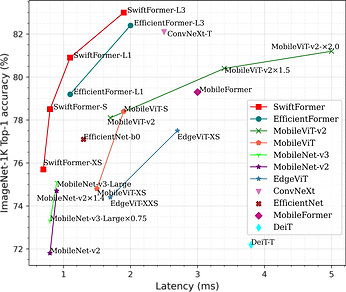
SwiftFormer is an efficient general purpose vision backbone for mobile devices. It introduces a novel efficient additive attention mechanism that effectively replaces the quadratic matrix multiplication operations with linear element-wise multiplications. Unlike previous methods, the proposed efficient attention design can be used at all stages of the network, enabling more effective contextual information capture and achieving superior speed-accuracy trade-off. The small SwiftFormer model achieves 78.5% top-1 ImageNet-1K accuracy and runs at only 0.8 ms latency on iPhone 14.
EdgeNeXt: Efficiently Amalgamated CNN-Transformer Architecture for Mobile Vision Applications | CADL, ECCVW 2022, Oral | | Paper | Code | Website |
Muhammad Maaz*, Abdelrahman Shaker*, Hisham Cholakkal, Salman Khan, S. Waqas Zamir, Rao M. Anwer, Fahad S. Khan

In this work, we designed resource-efficient general purpose backbone network for vision tasks. We combine the strengths of both CNN and Transformer models and propose a new efficient hybrid architecture EdgeNeXt. Specifically in EdgeNeXt, we introduce split depth-wise transpose attention (SDTA) encoder that splits input tensors into multiple channel groups and utilizes depth-wise convolution along with self-attention across channel dimensions to implicitly increase the receptive field and encode multi-scale features. Our extensive experiments on classification, detection and segmentation tasks, reveal the merits of the proposed approach, outperforming state-of-the-art methods with comparatively lower compute requirements.
UNETR++: Delving into Efficient and Accurate 3D Medical Image Segmentation
Abdelrahman Shaker, Muhammad Maaz, Hanoona Rasheed, Salman Khan, Ming-Hsuan Yang, Fahad Khan

In this work, we propose a 3D medical image segmentation approach, named UNETR++, that offers both high-quality segmentation masks as well as efficiency in terms of parameters and compute cost. The core of our design is the introduction of a novel efficient paired attention (EPA) block that efficiently learns spatial and channel-wise discriminative features using a pair of inter-dependent branches based on spatial and channel attention. Our spatial attention formulation is efficient having linear complexity with respect to the input sequence length.